Why MadKudu pivoted their company’s focus from churn risk detection to conversion prediction.
Francis Brero
|
CFO & Co-Founder
of
MadKudu

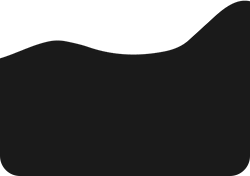
Francis Brero

Episode Summary
Today on the show we have Francis Brero, CRO and Co-Founder of MadKudu.
In this episode we talked about how Francis started out studying mechanical engineering and ended up founding a company now serving as the CFO, what MadKudu is and how they help their customers, and why starting off the company by helping companies predict churn didn’t work.
We also discussed how a typical customer uses Madkudu and what success looks like for them, how Madkudu predicts retention at different time horizons, and the three stages of a customer’s lifecycle every CS team should know about.
As usual, I'm excited to hear what you think of this episode, and if you have any feedback, I would love to hear from you. You can email me directly on Andrew@churn.fm. Don't forget to follow us on Twitter.
Francis Brero
Recommends
Reduce Customer Churn by 20% in 2 Days With Predictive Analytics and 3rd-Grade MathThe Assessment Every Business Needs to Do ASAPMentioned Resources
Transcription
Andrew Michael: [00:00:00] hey, Francis. Welcome to the show.
Francis Brero: [00:00:02] Hey Andrew. Thanks for having me.
Andrew Michael: [00:00:04] It's a pleasure for the listeners. Francis is the cofounder and chief revenue officer of MadKudu, a predictive engine that analyzes user Pavia to help predict conversion rates. Upsell opportunities and customer attention, prior to MadKudu Francis was the director of implementation and sales engineering, and agile one. And so my first question for you Francis is how did you start out studying mechanical engineering and ended up finding a company now serving as the chief revenue officer?
Francis Brero: [00:00:32] I think it's overall. The background that I had was doing a lot of different things. I started in a theoretical mathematics then went into applied mathematics and then some mechanical engineering at Stanford, at the D school, which I think was where I really got introduced to the concept of, entrepreneurship.
And especially that, you know how that concept is embraced in the Valley. And that led me to really want to join. I'm a startup I'm down here and that's how I joined [00:01:00] agile one. And, through my experience there, I, learned a lot going from. being a seed company all the way to series C a was like, rounds led by some of the most prominent, VC firms out there, the Sequoia Mayfield and Tonita's of the world.
and then at some point I realized as the company was, starting to hit the kind of 150 employee Mark, that I wanted to go back to, building something from scratch. And that's how, MadKudu started.
Andrew Michael: [00:01:28] Very cool. that's actually, I'm at a very similar point now, at Hotjar currently, I'm, it's getting to that point where it's a hundred plus now, and that's, you're getting that itch to get started again.
But, the one thing I wanted to ask and maybe it might just be, term that you were there, but I notice at Stanford, you were there for a year. Does that mean you were a dropout or does a specific course that you took?
Francis Brero: [00:01:50] I wish I could say I was a dropout, like every cool founder in the Valley. Yeah. so I studied in France and essentially.
My engineering school, allowed me [00:02:00] to do, a year exchange.
Andrew Michael: [00:02:02] Yeah. I thought maybe you just did the cookie cutter models that go to Stanford dropout on this. cool. And so I probably didn't do the best job of explaining what you do, or maybe you want to explain a little bit more detail. what is Madkudu? how do you help your customers?
Francis Brero: [00:02:16] Yeah, no, I think you did a really good job. I think the easiest way to explain it. It's really at the high, low, where, and it almost goes to the origin story of Madkudu, where one of the realizations we had at agile one was that we were a predictive analytics company and we were doing very little, analytics research on our users.
And, as a SAAS company, that's important to know how your customers are using your product, how that's evolving over overtime. And we realized that there was a really any good solution out there to do that. And to B2B space, they were some in the B2B and the B2C space. And so we, embarked on the route of trying to help companies.
Leverage all the data they had about their users and customers to be able to make the right decisions. And so today that's still the, the [00:03:00] lifeblood and mission of Madkudu, which is really helping marketers and, revenue teams turn their data into insights and leverage those insights to turn them in to revenue.
so it's really taking a pragmatic and operational, perspective on applying data science to user data.
Andrew Michael: [00:03:18] Very cool. and then you you mentioned as well in the beginning, I think that starting out with Madkudu, you initially looked to try and help companies with churn and analyze that, realizing an agile one.
can you elaborate on that a little bit? what was the idea behind it? Why did you choose predicting churn as a starting point?
Francis Brero: [00:03:39] Yeah. at the time the company was growing and we're looking at valuation and we're seeing, that, even just looking at the fundamentals of the company and realizing that, we have, an average deal size, which was in the, like the 200 K kind of range.
closing a deal is excellent. Losing a customer is absolutely terrible. and it's really bad when you lose a customer and you realize that, potentially they haven't been using the [00:04:00] platform in three months or haven't been using it the way you expected them to. So we realized that was a problem.
And we were trying to figure out if there were any tools that would do that. None, no tools out there would do it. And what we found doing some research, trying to find a tool for ourselves was that there were some really excellent companies out there. If you think of like the. So tango or Gainsight's of the world that really focused on that CS space, but from our interactions with some of their customers or people.
Yeah. People that had worked with those companies, those, companies are excellent at driving, operations and processes, but they don't necessarily. Excel and the ability to leverage high volumes of data to do pattern matching or identifying, any trends into data. And so we set on a course to say, like the best companies and the biggest companies in the world, they can afford to hire and pay data scientists to mine, the user data, to look for patterns that might indicate that.
There's lost [00:05:00] a loss of trust or that some customers might churn, but most of the companies out there just cannot afford to hire a data scientist. So how can we build something that connects into all the analytic systems that exist out there at the time? Like Kissmetrics Mixpanel segment amplitude pull the user logs.
And from there looked identify any usage patterns to be able to bring that up to the CS team, to help them identify that there might be an issue with, with one customer or another. interestingly, I guess the data science, part of that was very successful. Like we manage really well to identify.
when there were issues and were able to bring that up to CS teams. I think that one of the main challenges we found was that our customers were struggling was the operationalizing of this, model. It tells you watch out this customer is at risk of churning. We're seeing that their usage patterns have been affected by these two downtimes or by these little things here [00:06:00] and there.
And as a consequence, they're, daily usage has dropped by 30% or whatever. that's great information. It's helpful for, the CSM or the support team. If it's a distributed team to. No, that they should reach out. However, what often happens is that if they reach out and the customer is about to churn, there's something that's fundamentally broken, in the usage of the product, like they might have, there's something missing could be a feature, could be trust in the product could be reliability.
and that is something that is very difficult for a support or a CS person to fix. And so what we found was that very often though, we were able to identify customers that were at risk. We weren't able to find the right playbook to help the CS team prevents that churn. And I think that was probably, my assumption is that's probably what, the great folks at Gainsight and Totango have found over the years and why they focus more on building the playbook for the CS team to know how to almost prevent those [00:07:00] disruptions in patterns of usage and really how to have the CSM, Fight churn and ensure that the customer is getting recurring value from the product.
And I think that's what I've, that was the learning on that side. Is that the core, thing that is important, I think in, in retention and churn is really processes. Like the data can inform your processes, these, but you need to have excellent, operations from an execution standpoint, talking to the customer and getting them to see value.
Andrew Michael: [00:07:28] To be able to impact. Yeah, I think this is it's speaks to another issue as well. That pretty much anybody that really goes about trying to improve a churn and increase retention is typically, I'd say 80% of the time I've spoken to people. They start with churn first. and they try to see how they can avoid churn.
And they like you said, try and predict who's leaving, but more often than not. It's almost too late. if somebody made a conscious decision to stop using your product or to actually go into the product to churn. It's very difficult [00:08:00] to train and change that behavior now to win them back and to keep them going, obviously, central be able to save, but ultimately I think as well, what you're leading to is a, it's always going to be more beneficial as well for the business and for the company, really to focus on the.
What's driving retention. And how do you get more people to navigate that point? So almost in a way like a prevention is better than churn. how have you seen this evolve now in what you do at Madkudu and ah, like not only for your customers, but as an organization, how do you treat the two internally?
Francis Brero: [00:08:32] Yeah, I think so for us, that started a big shift. Where are we for the same customers? We started applying the same level of analytics to, conversion prediction. We're saying, okay, let's look at usage patterns in, free trials or in freemium models. And let's try to understand who is showing the right kind of patterns that indicate they're likely to buy.
And from that, let's actually trigger the standard cells outreach. sales is on the bright side is something where the. The process and the [00:09:00] execution of reaching out to someone to try and close them on your product is very well established, at an earlier time in companies. And so that ended up being extremely successful also from an ROI perspective, because we were able to say, we know we yielded X, many incremental conversions and therefore, we could say that we are in part responsible for the full lifetime value of these incremental customers that you wouldn't have closed without Madkudu.
Whereas on the churn prevention side, at best we were going to save, the subscription of a few customers for a couple of months. And so there was like a big Delta in terms of the. Incremental ROI that we could get from the predictive analytics. And that's why we ended up focusing on really the conversion aspect.
And I think that was my big learning. Is that looking at, maybe like earlier stage, if we're looking at series a series B companies, the go to market execution. from a sales perspective is generally at a much more mature stage than the, execution on the [00:10:00] CS side. And so therefore there's a bigger gap to be filled on, predictions for the go to market team, because they already have the executions to support more intelligence being brought to the organization on, on support.
And so that's where now, when customers are saying that they want to have. Churn models. We go directly into more of a consultative approach and try to help them put in place some good processes to, to leverage some of the data in very basic ways that can help them highlight customers that might be at risk, but we really stay away from the, the predictive element.
Andrew Michael: [00:10:35] Absolutely. Yeah. and that makes a lot of sense. I think the other thing you mentioned as well, was interesting concept is, or is that. Customers are companies that are larger, they can afford to hire data scientists to build these models. and you wanted to build something for those that couldn't afford.
I'd even argue though that all the times companies that can afford to hire data scientists, it's not always the best idea because, Building up on the knowledge and domain [00:11:00] expertise, texts time, it takes a lot of experimentation. do you see the specifically only for earlier stage companies or companies that can't afford it as a science, or do you see value in something like this working in conjunction with other debts?
Because I've seen as well, many times. Okay. The data sophistication of an organization is probably the most important in terms of how they can take advantage. a lot of this stuff you can, in some ways, Do it out of the box, like Kissmetrics and Mixpanel, and you could figure things out the way, but having something like Madkudu , I think that automates this function for you and shows you what you should be looking for instead of gives you everything and asked you to try and find what you're looking for.
I think for me, at least from the surface, that's what sounds like the key, like how do you see it internally?
Francis Brero: [00:11:45] I think. Yeah. and not to pitch against Madkudu, but I think especially on the CS side, the way I. I like to look at this also is, the difference in, in trading between high frequency trading and maybe, like longer term [00:12:00] investments, like hedge funds or whatever.
when you're doing high frequency trading and like each transaction is going to be at a much lower value. And so that's where you want to be able to react really quickly to any new change in the dataset. whereas when you're doing, more longer term investments, like your. You're really looking at a broader set of data points and slow moving points and trying to make decisions based on, like information that isn't necessarily digestible easily by a computer.
And also because it's going to take a long time before you see the results. And I see that churn is very similar to me to that, low frequency, trading, because, typically in B2B have annual contracts, so it's not you're able to apply a quick change, and monitor how that's going to impact your retention or your churn.
and so it's, yeah, the same logic applies there where you want to have people that really understand what's going on at a more macro level. there are things that you're not going to see in the data, It's if I know you're working with, a company that, you know, unfortunately, because of COVID has had to lay [00:13:00] off 70% of their team.
regardless of what the usage says, right? there's just like a bigger macro problem that is going to very likely lead to churn. And that's something that like a, a CSM should have expertise in how to deal with this and how to figure out how to work with the customer through these difficult times.
That's not something that the data is going to show you. So I think. while there, there is value in starting with like first principle, starting with like very basic, very basic approach of leveraging the data to do the right things. And then you can start going into leveraging things like Madkudu and say, okay, once I've done the kind of basic and obvious, I have the basics in place to make sure I check in with my customers often.
I know if they're using the product and trying to understand. Which particular types of events are going to be more important than others. That's when Madkudu can help identify that, but from what I've seen, your companies that need that are going to be at a much later stage.
Andrew Michael: [00:13:58] Yeah, for [00:14:00] sure. You mentioned as well, a couple of times about results from ad kudu and working with customers as well.
Sometimes you consult and advise them in different areas. maybe, can you talk us through, like, how does the typical customer use Madkudu? what would success look like for them?
Francis Brero: [00:14:18] Yeah, on our side, it is, it's interesting with AI. AI software has definitely like a big component of education, right?
So we have CSMs that, take care of our customers. They're designated for each customer. They take care of the customer from the, the onboarding onwards to understand what the business need is and therefore how the predictive analytics that are going to be configured for them will be, will fit into their, into their operations.
I think that's one of the. The biggest challenges is making sure that our CSMs are able to understand how having this better understanding of what a good prospect looks like. What a good prospect does. Having that [00:15:00] information can actually change operations in a way that's going to yield. The highest amounts of results.
And so the engagement with Madkudu, there's really two components. There's the core platform that's going to perform all these predictions. That's going to provide insights, but then there's the second part of, as I said, that's like turning data into insights. That's what the core platform does.
And at this stage of the company, this is where we then use the CSM say, okay, how do we use these insights to actually transform it into revenue? Because. Yeah, insides don't pay the bills and that's where the CSMs are going to be coaching the customers to make sure that they focus on, what is going to, give them the biggest bang for the buck and where, they'll have the highest impact.
So we might find some insights that are really interesting, but affect a very tiny portion of the population. So making sure that we don't get customers to dive super deep into that, but rather to start, putting something in place, that's going to have a higher impact and also. Teaching them that you always want to apply the principle of crawl walk, run. [00:16:00]
I think especially in the world of data analytics, people have all read about regressions, new neural networks, all this kind of stuff, and they're, they understand it just well enough to be dangerous for themselves where they want to go deeper. They want to understand more or about how it works. But that becomes detrimental to the actual utilization of the insights.
And so that's why really focusing our CS team on pushing people to execute, rather than, trying to achieve a perfect model is a big component of, our engagements today.
Andrew Michael: [00:16:31] Yeah, not to fall into the analysis paralysis trap and trying to seek, I think that's definitely something we've been trying at Hotjar to drum into sort of the team is really about get something, working, MVP mindsets, and the models we develop or whatever we work on in terms of analysis, it really needs, what can we deliver today to provide value?
And then. Think about how can we improve it tomorrow as well? It's a trap you can easily fall into when you hear all the buzz and the, you read the [00:17:00] books, which are,
Francis Brero: [00:17:01] and I think that the problem is in these, no one wants to do. Nuanced anymore, right? just look at political debates, whatever you want to pick.
Nobody is interested in something that's nuanced. We want, either very black, very wide, it has to be like polarized. And I think one of the challenges with data analytics and predictive models is that it's, there's a lot of nuance. one of the most famous statisticians, Mr. box that said, he wrote this like in one of his papers, like a very famous quote.
He said, all models are wrong, but some are useful. And his point was that a statistical model. And is that one thing is to create as accurate as possible of a representation of the world, but it is a representation. It's always going to be wrong to some extent, but really why it is designed is. To make use of it to drive some decisions.
And so we should focus. It's more on the usefulness of the model rather than then the accuracy, because we're never going to [00:18:00] achieve a hundred percent accuracy, but that is something that is very hard to understand. And so not just understand, but really to be at peace with because we all want to see, the, the deep learning network that's going to understand from looking at YouTube videos, what a cat is.
the truth is that in most cases it's actually going to fail a little bit. but then again, like what's important is can we, recognize the contour of a face? Can we recognize the contour of a dangerous object? That's falling to drive the right action. That usefulness is what we should focus on rather than our ability to build, one model that's going to be able to operate beta better than a human brain and understand everything.
Andrew Michael: [00:18:39] Yeah, I think in these cases as well, if there's another good quote by Ronald Coase, I think it was like, if you talk to the data long enough, it will confess to anything. There's always going to be different ways. You can look at data, always to getting different interpretations that can be made as well.
So it's not only about. not having all the right answers and sometimes being wrong, but also who's reading and looking at the [00:19:00] data as well. So I think definitely from that perspective, it's really important to ask yourself when I'm analyzing and trying to make decisions off of data is What do we, what happens if this is wrong?
And I think that reversible door concepts that Jeff Bezos talks about a lot is that you don't need to be right all the time, as long as like they're not irreversible decisions. So having the data and having something actionable is always going to be way better than spending the time to do months and months of research and analysis, trying to perfect.
Any model that you work on. I think that's why probably leave it up to the Gaza mad kudu to help out with as well. So you can get focused on other work that delivers value. cool. So one thing I'm interested in asking as well is, what's one thing that, you know today about churn and retention that you wish you knew when you got started.
Francis Brero: [00:19:52] apart from the fact that yeah, operations are more important than data prediction, I would say it's probably the. One of the, [00:20:00] I guess like secrets that we figured out was that, it's easier to build models that predict retention and use them to predict churn than to try and predict churn, as the outcome.
because when you're looking to predict retention, what the model is going to start identifying is what are the behaviors that correlate to getting value from the product and really what. What matters as long as someone is getting value from the product, there is a high chance they're going to be retained.
and really the, the group of people that you should be worried about are people that are not getting that value from the product. And so marking those as the people who are likely to churn is generally a better, a better way to look at it than to try and. and run churn predictions where you're going to look for huge things and patterns of someone going from no, I dunno, like having a, whatever 30% drop in usage or something like that, where if they're still over the bar of, of, you know, break even in terms of [00:21:00] like cost to the value they're getting, then they're probably not at risk of churning.
It might just be some macro event that's happening. Yeah. Traffic on their website or whatever. So I think that was really a big one for us, was to start looking at how do we predict retention, at different time horizons and how do we use that actually to predict churn and to start setting milestones that we want people to hit.
And that's really when we started building that, almost like decision tree of if people are on the right path, that's great. Keep on pushing them. And as soon as they fall off of that kind of like happy path standard, branches, then that's when we want to have someone intervene.
Andrew Michael: [00:21:39] Interesting. Can you dive into that a little bit more detail?
So you mentioned like at different horizons and looking at retention and seeing where they're at, like, how is this working? What are you looking for? What is the horizons and timelines that you're looking to see a certain behavior by?
Francis Brero: [00:21:54] So from, when you look at the data, there's generally a couple big inflection points in the, in [00:22:00] cohort retention, right?
So again, it really depends on how. How your go to market, is positions. And it also depends if you're on, monthly payment, quarterly, annually, whatever, like the lens of your contracts. But typically you're going to see that for a given cohort. if you look at that kind of cohort, like pure retention, removing upsells, or just from a like NetLogo perspective, you'll see that there's like some inflection points where there's like big chunks that, that ended up churning.
And so what you want to then do is to say, okay, we want to build retention predictions around those different timeframes and figure out what is important at that, around that time to make sure people are retained. And we often see that, yeah, there's going to be different importances if take a very simple example.
So we have customers who will have, like opt-outs often 90 days, of a free trial or whatnot. And the onboarding there is going to be extremely critical. Making sure you have. Let's say if you're a [00:23:00] CRM, Making sure that you've connected your Gmail, you've loaded contacts, you're creating deals.
You're closing deals, like making sure you've at least closed one deal in those first 90 days is going to be incredibly critical to make sure that you're retained. Because if you're not closing deals, then you're probably not seeing yet, the value from a CRM. that you're paying for, however, loading your initial contacts or connecting your calendar or your Gmail is not something that matters when we're looking at, a year past your initial conversion and trying to predict if you're going to be retained.
Then the question is really are do you have, Have you invited more people, are those people logging in? What's your total number of users? what percentage of your go to market team is actually logging in to that CRM? Those kinds of events become, a lot more important.
That means that you also need to design the phase, between the first 90 days to the annual renewal to incorporate things that are gonna increase that stickiness.
Andrew Michael: [00:23:57] Yeah, so it sounds as well, there's sort of [00:24:00] two distinct phases and the timeframes and horizons can change depending on your product and your customer base, but, the activation phase, which is that first initial period where depending on your product, it might be 30, 90 days or whatever it is, where you see maybe potentially the biggest drop off in churn.
And during that period, it's really looking what are the key actions that users are taking to get set up, to get that value and actually getting that value? if they don't get that value, that's going to be lucky key indicator that they're not going to be around. And then the second phase I think you're talking about is going more like.
The depth and looking at engagement and seeing, okay, what are they doing to increase their engagement in the product? If it's depending on the product, is it inviting new team members to their account? Like you said, is it potentially, increasing that intensity or the frequency of usage, which will indicate and give you a better idea of if they're getting more and extracting more value.
Is that correct? In saying like you typically would maybe look at two different phases, one being activation and the second then being engagement, when it comes to these predictions,
[00:25:00] Francis Brero: [00:24:59] I might break it into three and think of three different stages of the life cycle. And I recommend every CS.
Team to have that kind of mapping, at least internally and their CRM or customer, a tool to understand where people are. So I usually break it into there's an implementation phase. There's a ramp phase. And then there's the cruise phase implementation is when you're really getting things set up.
So you're connecting all of your integrations, just getting the tool up to a place where you can start using it. And until that implementation is done, Zero value is going to be obtained by the customer, right? It's really just technical setup, getting everything right up and running. And the length of that phase is important because that's pure cost, right?
From a, from a customer perspective, there's like costs for the software there's costs of not having the tool. And just so the longer that goes, the longer you are in that. you're just burning that, like CS cash, if you will. And then there's the ramp phase where now you go into, okay, how do we drive adoption and start getting the [00:26:00] initial value from the product and start growing that, adoption within the team.
And then cruising is once you're in cruising mode, everything is set up. People are using the product. There's going to be some more team members that get added as they get onboarded to the company. But overall, like the main use cases are identified. No, there's ongoing value.
that's been hit. and that's yeah. That's something that ideally, you want to achieve, three quarters before a renewal.
Andrew Michael: [00:26:28] Yeah. Very cool. So thinking about it in those sort of three phases, and then really try and look at from a routine perspective, what does everybody need to do to hit and achieve and get to those different phases in their journey?
I see we're running up a little bit on time, so there's a question I'll ask every guest that joins the show. I'd love to hear your opinion as well on Francis is let's imagine a hot pathetic scenario now that you joined a new company. And when you join this company, general attention is not doing great.
the CEO comes to you and asked [00:27:00] you to try to turn things around for them, but he's on a tight deadline to try and get results and needs to see something happen in the first 90 days. What would you want to be doing with your time to try and make a dent?
Francis Brero: [00:27:14] Yeah, it's a great question. I would probably try. so again, it would depend on of course how, contracts are set up and how churn is happening, If If we're on annual contracts and no opt, then I'm making sure to talk to every single customer that is up for renewal in the next, I would say three to six months, if then if it's like monthly contracts, probably talking to as many customers as possible to understand how they're getting value from the product, if they are, and starting to.
Yeah. to monitor, to monitor. Yeah. Just to classify our customers in terms of, are our customers getting value from the product? Yes. No. And then going through probably yeah, just interviews to try [00:28:00] and figure out from them what's missing and then take it from there. I think it's, it w it is difficult to there's.
I don't think that recipe that can be applied of Oh, if you do this, it's going to work. I think it's really a matter of reaching out to customers and understanding how they're getting value from the product or what's. Prohibiting them from getting that value.
Andrew Michael: [00:28:19] It's very interesting that you mentioned, like religious speaking to customers first, as opposed to opting and starting to take a look at analytics and data and analyzing.
and I think it's something you alluded to earlier though, as well as like, when it comes to general retention, the work that you do today is only seen tomorrow. It's not something that's immediate and, The impact that you're going to have a potentially, like you say, from having these conversations face to face direct is immediate and it's felt as opposed to starting from the standpoint where you're really looking at the data, trying to understand, trying to fix things.
If you're not having those conversations, you're missing all those signals. You're missing that. the company retain 70% of the people, like you said, and, they're going turn anyway. You're missing all sorts [00:29:00] of other things that might. In the way that you can really just get a better grasp of understand, can inform and influence, how you go about approaching it.
Francis Brero: [00:29:07] And again, it's a question of maturity, right? If you joined Spotify and they tell you, Oh damn, we're having, bad retention numbers. And I would assume there's probably an issue somewhere in the platform. And that's where you can start looking at the data a little bit and potentially still talking to customers.
Cause they, they might help you figure out a hypothesis quickly. But generally the other issue with. predictive is that if you're not tracking it, then there's no way you can use it. You can use that to predict anything. And very often, people are having trouble with, with a product because, there could be a missing feature there's no way to track that there's a missing feature.
So sure you could, open up boards for customers to request features and things like that. But at the end of the day, people don't necessarily know. How to call what it is they need. it's the famous again, like just to use quotes, but like Henry Ford saying that if you listened to people, he would have [00:30:00] given them a faster horse.
Like ultimately they just want it to go faster from point a to point B. and if you listen to customers, they're just going to present their needs or how they want their needs to be fulfilled within the scope of what they under. And that's where that's kind of customer discovery is critical to. build empathy with the customer, understand what it is they're trying to achieve, where you fit in their success within their organization.
And then look back at the product to see where you might not be helping them achieve that full potential.
Andrew Michael: [00:30:35] Absolutely. Yeah. I think this is also one of those things where talk about, in business intelligence, at Hotjar is. A lot of the times like analysts can really get stuck in, wrapped up in crunching numbers and analyzing data.
But if you're not having conversations with customers as an analyst, you have a very big blind spot in terms of the ability to empathize and understand what they're going through, what are the problems. And it will make you a better analyst as a whole, [00:31:00] as well. Being able to inform maybe some of the decisions or areas that you look into that.
I can say, you're not going to get the full picture from just looking at data and having those conversations, being able to empathize, being able to understand, your customers. Nothing can be that.
Francis Brero: [00:31:14] Yep.
Andrew Michael: [00:31:16] Cool. like we, up on time now, the sort of final thoughts that you want to leave the listeners with the new thing they should be aware of or how they can keep up to date with what you're working on at Madkudu
Francis Brero: [00:31:27] of course I'd recommend them to subscribe to our blog at Madkudu.com just because I think we, there's some fun analyses in there and we talk a lot about data science and how to be pragmatic about it. And we do have, a couple of recipes out there on how to build your first kind of churn model, if you really want to build something yourself.
so I definitely recommend checking that out and reaching out if they have. any questions on how to get started, how to do it, or if they re you know, would like to see any updates to that kind of information.
[00:32:00] Andrew Michael: [00:31:59] Very cool. thanks very much for joining the show today. It's been a pleasure having you and wish you best of luck now going forward.
Francis Brero: [00:32:06] Yeah, thanks for having me.
Comments

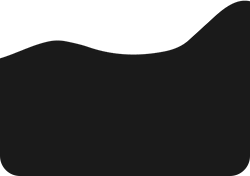
Francis Brero

A new episode every week
We’ll send you one episode every Wednesday from a subscription economy pro with insights to help you grow.
About
The show
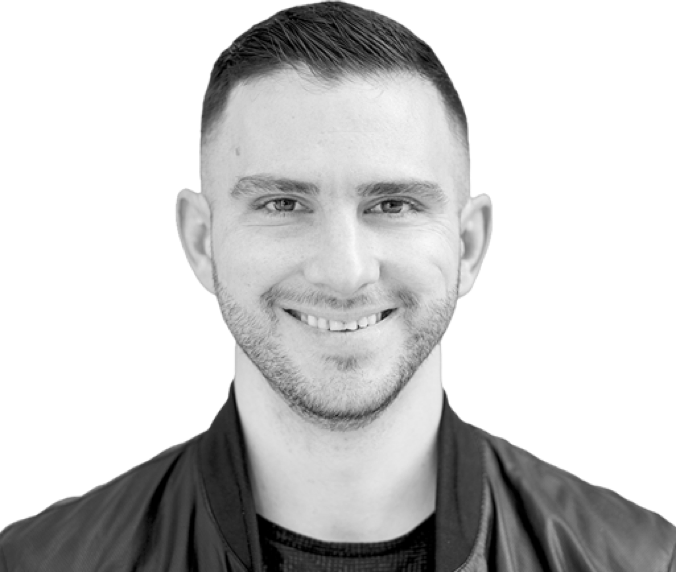
My name is Andrew Michael and I started CHURN.FM, as I was tired of hearing stories about some magical silver bullet that solved churn for company X.
In this podcast, you will hear from founders and subscription economy pros working in product, marketing, customer success, support, and operations roles across different stages of company growth, who are taking a systematic approach to increase retention and engagement within their organizations.