Why you should rethink churn exit surveys and what to do instead.
Tadas Labudis
|
CEO
of
Prodsight
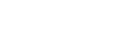
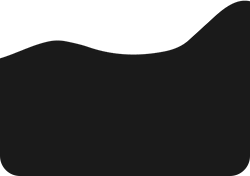
Tadas Labudis
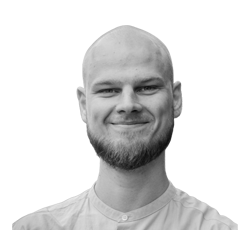
Episode Summary
Today on the show we have Tadas Labudis, co-founder and CEO of Prodsight.
In this episode, we talked about how multiple channels and siloed feedback can be a company’s biggest customer feedback analysis challenge, and how good data hygiene is the foundation of data collaboration across teams.
We also dove into why you should rethink your churn exit survey and what you can do instead. Lastly we discussed the different ways you can analyze churn against support tickets and feature requests.
Mentioned Resources
Transcription
Andrew Michael: hey, Tadas. Welcome to the show.
Tadas Labudis: [00:01:28] Andrew happy to be here.
Andrew Michael: [00:01:29] It's great to have you for the listeners. Tadas is the CEO and founder of prod site, a customer feedback intelligence platform, which helps companies deeply understand customer feedback across all channels.
Tadas has previously held roles as a product manager at Javy and KubeCon and was the co-founder of even thread. So my first question for you to tell us is what is the biggest mistake in companies make when it comes to their customer feedback, besides the obvious answer of not paying attention to it?
Tadas Labudis: [00:01:56] Yeah. Once you pass that stage I think the next [00:02:00] challenge that a lot of companies face is it's siloed feedback analysis. So you might have different teams looking at different sources. You might have a different people having preference towards certain types of research methods.
For example, in a UX team, you might see people enjoying doing more in-depth interviews to, by doing five or 10, and then try and draw insights from that. And you can draw insights from that, but that's not the goal itself. On the other hand, you might have a support team that's processing 20,000 tickets.
So if you compare the volume of the data you're having here in signals, you could be tracking versus here and then let alone, looking at your view, start coming in, or people shouting you it's better or surveys that other teams are running. see like different teams having preferences, looking at different things, but not necessarily always developing that holistic picture of what's actually going on.
Andrew Michael: [00:02:52] That makes sense. I think obviously, like you said, it's different practices and different purposes when it comes to the top of feedback that you're looking into and trying to understand [00:03:00] how do you see teams working well together then across like when you've seen companies do this well, what are they doing to ensure that they're getting a good, broad picture when it comes to their customer research and balancing their customer feedback input.
Tadas Labudis: [00:03:13] Yeah. So the first foundation of collaboration with data is having good data hygiene. So that means recognizing the data sources that you have available where are customers reaching out in some cases consolidating. If you have three different channels for customers to reach out, maybe you could use one or use an Omni channel solution that kind of brings that together.
So once you have that, they didn't place and, depending on the size of the organization, The maturity, you might be talking about something as simple as a spreadsheet. If you're early on, maybe you can manage it there all the way to having a complex data Lake with a BI system on top of it, but just recognizing the sources and having that accessible to people and ideally in one place or two places as possible.
And then the next step [00:04:00] is having systems and processes for regularly. Reviewing and analyzing that data. So it's not to say that you need to analyze each interaction or each piece of feedback, but having an understanding of update idea of sampling. So if you're sampling feedback, it's a good idea to sample across the range of sources because each source could have its own slammed.
For example, if you look at support data, that's going to be very much driven about what's wrong with the product or what's wrong with user education. That's not going to tell you much about prospective users because they haven't gotten to the point where they can raise a support ticket. If you look at a survey that you're running, but a specific set of questions, that's going to have answers to those questions, but might not reveal much about what current customers are struggling with.
So if you have these different collection points across the user journey by combining them. And, or at least sampling each of those. Yeah. Same time and [00:05:00] revealing your insights will allow you to have that holistic view. So th that, mature companies or companies that need to care about feedback and doing well, they have these components in place, but of course there's much more to it.
Andrew Michael: [00:05:11] Yep. So there's a lot to unpack here as well before, before we do that though. Maybe you just want to give us a little bit more of a clear overview of prod sites, like what it is that you do exactly. And how are you helping customers? Cause I think a lot of what we're going to chat about is going to revolve around this tonight.
Tadas Labudis: [00:05:26] Yeah, that's a great question. For me, proxite comes from, my, my own role as a product manager is working on a mobile product. And I was in charge of managing that feedback. We didn't have the luxury of having researchers and just had to make sense of that data ourselves.
I just kinda started building processes internally starting with the spreadsheet, then air table came along and it's trying to be sophisticated and SAPs and things like that. But at the end of the day, I said I'm starts growing. All this process started breaking down. I connected my passion for.
Entrepreneurship, I launched a small startup at the university. It wasn't, a big success or anything like that. [00:06:00] And that's why I into the product management to learn from other people and grow. But always had in the back of my mind, like if I find an idea I'm passionate about I'm going to come back to that, launch something again, and that just collided into this opportunity.
And I decided to build a system that allows people to understand their customers. But at scale and data volumes grow. And in terms of what ProSight does, it think of that in three steps versus getting that data in the same place. So we built bridges with a lot of different systems like intercoms and desk Trustpilot app store reviews, and so on.
We bring that together into one data step. And then when you've run a range of different types of analysis on top of that data set in consistent ways. We do sentiment analysis, level of emotion or people, angry about or happy about what's the intent that they haven't, they reaching out our date to talking to you, sir, to compliment you about something.
Our, the conduct needs to say that they want to churn or to request the features or report a [00:07:00] product issue. We can detect those kinds of things based on an algorithm that would be developed. Based on customer data. And then the third piece is what exactly are people talking about already mentioning different features that you have in your products, ask them questions about billing, pricing and so on.
So understanding all those components and putting into our real-time dashboard allows us to give a time view of what's happening to customers. What matters to them? What's creating friction. In their experience and allowing it to slice and dice that data in a way that allows us to make changes.
Andrew Michael: [00:07:38] Very cool. Just giving like teams, the ability to centralize all their user feedback from multiple different channels that it's coming through, whether it be like direct feedback forms that you're looking for or in the form of like support tickets or feedback on review sites for my understanding.
And then on top of that, essentially you're classifying running different algorithms to be able to give. Quality [00:08:00] almost. So you're quantifying qualitative feedback and then giving your users the ability to make informed decisions based on a broad set of feedback coming in from multiple channels.
It's very cool. The thing I wanted to do touch on a little bit, as well, as you mentioned, it's in the beginning, I think is making sure that you have a clean data set and bringing information in. Cleanly. And I think this is one thing I've always found, like to be super effective when it comes to things like surveys or it comes to any sort of trying to quantify any sort of qualitative feedback is having a really solid understanding of the segmentation that you're going to be wanting to do.
From the beginning which really helps you enable you then in the long-term to be able to break that data down and CN, an example, being in a survey, you might ask a few questions to begin with around like demographic or fermographic data. So what is your role? What is that your company and so forth?
Having that standard is, does across all your different channels really helps you then be able to break it down and look at K, show me all the feedback [00:09:00] from product managers or anything like this. Is this something that your product helps companies do? Is this something that you work on with them in terms of setting best practices within the organization?
Like how have you seen companies handle this really well? Where they have a really good system that scales and enables them to segment their feedback effectively?
Tadas Labudis: [00:09:16] Yeah. So I think when you are looking at feedback, that's solicited as this, the feedback you requested in a form or survey absolutely.
That's the perfect practice to have that qualification to top, so he can relate to the answers to a certain segments of your users. However, the majority of the feedback that we help our clients analyze tends to be unsolicited. In my view, a support ticket that's raised. It could be about anything and it doesn't have pre qualifying questions.
It's more of a conversation treads where the Asian DCers interacting, or it might be online reviews where someone is just a volunteer to go on and it gives her a feedback and some systems do have questions that kind of structure that it's you know what's the value received from this product?
What could be improved? [00:10:00] But sometimes the reviews are just a single block of texts, like on Trustpilot. And there's not much context there. What do we do with those data sources where possible is try and add structured data that relates to the attributes of the people that raise that. So if someone raised a support ticket and their user, or based in a certain location, they might have a certain plan associated with them or certain MRR values or any attributes that might be relevant, be sink dose into our system and then expose them for filtering.
So you have access to the whole user base of what people are talking in general. But if you want to understand specific segment, you can use those filters to control down and look at what is creating the most friction for your enterprise customers. For example,
Andrew Michael: [00:10:47] Yep. Absolutely. I love that because I think not like I'm a very big fan, believe that not all feedback is equal.
And it's really important for you to understand like who your ideal customer is that you're really trying to target and having them, the ability [00:11:00] to segment a feedback base of that, to make sure that. When you are looking at the quantified qualitative feedback that you're getting the right signals, and it's not just you might be getting way too many support tickets from the wrong type of customer.
And then you waiting feedback unevenly against him, just because of the volume versus actually like the business impact. Like you say, having something like MRR attached to it really gives you an indication of the fits for the customer, or potentially even maybe total man subscribed, just having another fallback to really understand.
Okay. How can we wait this feedback to make sure that it is going to be valuable for us to take into consideration? So let's dive in a little bit. You mentioned churn surveys. Churn feedback being one of those components and obviously due to the nature of the show there's certainly, I'd love to dive in today and see from your experience, working with customers, like what is some of the best practices that you're seeing people employ to really understand their user feedback and to see how they're actioning that then to reduce churn and increase retention.
So [00:12:00] maybe like we can start with a broad question what are some of the interesting things you're seeing with your customers and how they're using your tool to reduce churn.
Tadas Labudis: [00:12:07] Yeah. So one of the patterns I would like to address first is what I see a lot of companies do. When you look at the intersection of churn analysis and some kind of feedback collection about turning and understanding why it turns happening.
So I noticed that a lot of companies have these churn surveys or follow up surveys where basically someone churns. And then it's like, why are you turning, please fill out this form and then they'll cancel your account. And there's like different levels of hostage type situation at that point. Some were optional, some are mandatory.
So I think there's something wrong with those surveys. And of course, if you can capture that intent at that point in time, it's great. But what typically happens is at the point of cancellation users are already out of the door. So you don't have that Goodwill to give you. High quality feedback.
They might provide you with a quick answer. [00:13:00] They might like type in some random characters. So you're not always going to get the best feedback because they're not incentivized to provide at that time. Another thing that we see is in some cases, the person canceling the account on the actual UI is possibly someone from finance rather than the end user.
So they might not even know the exact reason why the company's canceling. So this is an assessed situation. You might not even be able to capture that feedback from that person. And in other cases, if it's a larger organization, there's multiple users. People don't even know, maybe they haven't reconciled by exactly.
They're not adopting your solution anymore, or why it's no longer needed. And they just know that they're not using things that cancel it. And then you're not going to be able to capture anything. So it might feel like a success to maximize or optimize for having as many surveys filled out or be them as detailed as possible.
But I think that's slightly flawed goal. And when we see some more customers, of course, that's biased because we see what people do with our product and it informs us it doesn't [00:14:00] necessarily give us a holistic picture of the market. But before customers, churns are tons of signs already.
If you're asking me what I'm turning today, chances are already left five support tickets, reporting, product bugs, maybe progressed some features a few times, maybe even less to review notes for improvement. So it's weird. It's almost like you ignored everything. I said up to this point, and now you're asking why I'm churning.
And I have I'm almost angry. I don't want to give you any feedback because you're not listening. Companies that we work with. We see them recognizing that they have to stay out ready. It's sitting there. You might be six months old or four months old by that time, but it's there.
So if you string along that journey of the customer from the beginning, or even pre-sales all the way to the point, they churned and filter down on that segment of trim users, you can actually find. Those signs that could be turned indicators. So typically, it's product deficiencies or product is not doing what it's supposed to do, or the quality of the product's not [00:15:00] good enough.
And that's going to be like a long tail list of things from things that are frequently mentioned to things that only a few users mentioned. So if you understand every bug report that every churn customer has raised in the last six months, You will have like a top five or top 10 list of things to fix.
Other areas that we see are feature requests. So the product might be fine for what it does, but it's just not growing as fast as the end user needs it to grow. And maybe there's competitors that are further ahead. So that kind of creates friction as well. So if you understand this feature requests are coming up.
Across your feedback you can prioritize those things and understand which ones are strategic features that you need to start building. And the last theme, our nature off of churn indicators we see is about product adoption. So here is the features might be there. The Prague might be high quality, but I just don't know how to use it.
Exactly. So it's user education theme. If you understand, [00:16:00] especially on the support side, all those different questions that people are asking at different parts of their user journey, then you can better address them upfront in the product or improve the usability of the product. So those questions don't get asked.
So it's I guess to summarize that it, what we see being a kind of a better process is going away from a small survey. Or you maybe capture some reasons in a term survey to looking at it a much more holistic, bigger data set of data to the customers are leaving as they're interacting with your customer service or your product.
Andrew Michael: [00:16:34] Yeah, I really like that point as well. And I think just going back to what you said in the very beginning is that at that point you're very lost the customer. They're already not in a great mood, like the type of feedback that you're going to get it for me, if those sorts of exit surveys are more quantified feedback that you're getting is just saying, okay, the general themes and reasons why we seem like we're not going to expect somebody to fill out a form and give us the reason why they're leaving, but just to hit a checkbox and say bugs or.
Price [00:17:00] or just to understand the general themes, but I really love the point as well of you making, being able to go back and look at their customer feedback history and seeing what's been submitted. Are there in terms of support or so boards have feature requests and then understanding from there, like what went wrong over the last six months, having that ability to actually look at like churn customers recently and look at their support tickets together.
It's not something easily done. Typically with technical sexy companies have, but. Doing it, I think can be very powerful. It also reminded me of actually something we discussed in a previous episode. And I still love this example something that David Darmanin taught us at Hotjar and came from his experience at conversion rate experts was.
The concept of not all feedback is equal mentioning this earlier, is that taking the example of a website, typically like a big mistake companies make, and this is a similar analogy to the churn exit surveys that typically what they do is they will ask the question like what could we do. To get you to convert today.
So they're trying to preempt some decision and [00:18:00] typically why this is very bad is you have normally three types of users. And I think they had a star Wars analogy. I might screw this up because I'm not a big star Wars fan myself, but there are three different types of users and it was like two buckers stormtroopers and there's a third type.
And essentially Like your groups of users fall into these three types of ways. These three buckets, the two buckets would be people that would just come to your site would just be browsing. Totally lost, would just end up leaving and not doing anything anyway, because they were never going to be your customers to begin with.
You have the storm troopers that were. Always going to be your customers, no matter what they were just going to come in stomp through buy your product and get done. And then you have the jetties, I think there was it's the jetties. And that would be the ones that weren't too sure. Like they'll come to your site.
They were checking out, is this for me? Isn't it for me. And then they'd figure it out. And the problem asking for feedback too soon is that you get mixed signals and you get feedback from these three different groups when you only really want to be getting from other, the stormtroopers or the jetties.
And probably the jetties being the most valuable because. They're the ones in between that [00:19:00] if you figure it out for them, what you can turn them into a buyer is really important. So what they realized conversion rate expert, actually the better question to us instead of what was like what would make you buy today was after purchase immediately after purchase asking what nearly made you stop buying today?
And that's why they knew that they were only getting feedback from storm troopers and from eyes. And then they could understand as well from the jet art's perspective, what were the things that they could be doing to improve their checkout process, the flow. And we discussed this on a previous episode where I mentioned, cause I think it's really interesting in the concept of.
Tronox surveys that you mentioned in the sense that we send out the surveys once people have churned. And like you said, in the beginning, like they're just not in the mood times to the system. Information is probably not super useful. Like the methods you mentioned like a hundred times, a thousand times better, and it'll give you much more fruitful results, but.
Asking a question to a customer who's just renewed saying what nearly stopped you from renewing today. Could almost be just as powerful as well. And you more likely to get a response because obviously the customer has just renewed with you. [00:20:00] They've just continued their subscription. Typically. I think this will work better on annual subscriptions.
Monthly might be a bit more of a risk. But I think the feedback you'd get from a survey like that would be much more powerful than knowing that you're actually getting from the right types of customers. And then seeing which ones that had renewed, but maybe weren't a hundred percent happy with doing it.
They just needed to do it. So they just didn't have the time to look for an alternative solution or event probably next time round, you would have lost them. So I love the point that you made on that. And I'm interested to hear your thoughts. I kept seeing feedback and this, like, how does that sound to you as well from your experience?
Tadas Labudis: [00:20:35] Yeah, I think asking questions that, yeah, I think you're absolutely right. You need to ask the question in a way that's going to give you the response you want. And sometimes it'll be default to the types of questions that are leading like this one about, what would make you stop buying today?
That's just rethink it. Wouldn't ask that normally. It takes some thinking and many ask that question. What would make you [00:21:00] stop buying to them and why you almost didn't buy today? It's makes the user think about the steps would they would need to take, or the buyers, maybe they need to get approvals.
Maybe they need to build a business case is thinks about what steps do we need to do as opposed to coming up with some kind of feature idea for you to add that would make it that much sweeter.
Andrew Michael: [00:21:20] I think it's because as well as humans were not very good at predicting the future, but we're really good at retelling the past.
So if we can retell our past experiences, it's much easier for us to do that then to predict like what we would have wanted or what would have happened. So that's something I definitely learned to one of my first startups, like terrible mistake of asking leading questions and then. Just getting useless information back there.
To that time, we thought it was amazing. Oh, we've got this great product. People love it. Everybody we ask is super positive. But if you look back at the question, we asked them, like it was a no brainer that they were going to love the answer to the question we were asking. Interesting. Let's go back then as well to the idea of bringing [00:22:00] together feedback and looking at recently churned customers and seeing things like support and seeing things like feature requests, how are you seeing companies doing this effective today? So instead of the notion of focusing on churn exit surveys, like we agree it's not the best place to look for insights from it, but how do you see some companies doing this really well in terms of analyzing churn based off of other support requests for feature updates?
Tadas Labudis: [00:22:25] I think there's. There's a few ways of doing it. And it kinda depends on what you can commit to internally and what kind of technology you want to purchase to help you along with that, it's like bicycle versus a car, different technologists help you achieve similar goals.
Of any many, you just start fundamentally at analyzing your data. So I think first of all, is to recognize that you have data coming in. On one hand, you have surveys where you're like praying that you're going to get responses. On the other hand, you have a fire hose of data coming in already.
[00:23:00] So it makes sense to start looking at that at least, and then seeing what are the remaining questions you still need to answer. But doing that logistically is quite difficult. If you have large volumes, so analyzing hundred responses by hand in a spreadsheet, doable. Analyzing 10,000 tickets, not so much because of the time it's going to take.
And then you're wondering is it going to be war? I didn't say I was going to be that good. So on a support side and that's the reason I mentioned support is because that's a data set. That's historically been hardest access for different teams. It's owned by the support team support department sometimes treat it as a cost center.
So it's it's not going to bring me good insights, but I think companies now are realizing that's a valuable source of data and that's something where it customers volunteering a lot of time to some is feedback. So what do we see teams do is agree on a tagging taxonomy and say, okay, we have the spending categories.
Anytime it requests, any of those categories comes up, [00:24:00] let's tag it. And that's training the agents to understand that solemnly exposing that in actual support system, UI and loss support system support, that kind of feature. And then at the end of the month or a week, you can look at the tallies of these things and say, okay, out of 10,000 support tickets, 1000 word about bugs, the problem is.
If you send that to the product team, that's not specific enough. Okay, I know we have box, last month we also had been present of tickets about bugs. It's like what exact bugs are causing issues. And then the problem you have is okay, it's 14 could identify maybe five most common and add it to taxonomy, but that makes agents think a bit, they have to think about even more categories.
And then what ends up happening? So you have these catch old categories that start aggregating stuff. So you might have five specific books and then bug other, and then [00:25:00] people just come default that if something doesn't fall into that category neatly, and then again, you back to square one. There's been a tremendous amount of development in the space of natural language processing and understanding, and there are machine learning.
All of those, there are. Different techniques like topic extraction, you can actually analyze texts like you would analyze quantitative data by running, scripts on that data. And that's what we've done. We've basically taken the advancements in the space, build some more proprietary stuff on top to to fit this use case.
And we just decided to analyze these data sets that are hard to access for teams. All of a sudden been an automated system go from broad categories to very specific categories because to an algorithm, it doesn't matter if it's 100 themes or 200 themes, you get more specific drill down insights.
And another thing you can do this, you can do it very consistently because human doesn't need to intervene. And assuming you've done your training correctly [00:26:00] is going to detect all those teams. Automatically in real time. And another thing we see is in one ticket or support interaction, you could have multiple different topics being mentioned.
Maybe it starts off with the question about how do I do this and then, Oh, it would be nice if you added this feature and, Oh, by the way, the screen crashed for me. You might have three or four different things being mentioned that are distinct and interesting to different teams, but. An agent will only apply one label because that's just one field to fill out.
So you're losing like three quarters of insight from that one conversation. So there's a range of methods and there's tools out there. There's also manual methods. And I think what's important is actually recognizing that the state has valve and starting to learn from it. And then how you do it as that's
just up to you.
Andrew Michael: [00:26:49] Yeah, that's very interesting. And obviously, like you said, there's different tools in the market. I think we'll obviously have show notes for guests to check out prototype, but I think the one thing you mentioned, [00:27:00] and I love it's actually something we chatted about recently with Mariah, from help Scott's is that this notion of luck support being a cost center.
But really if you use your support effectively being the frontline they introduced the concept of like support driven growth, where it's not just about supporting requests, but also like you have interaction points with your customer and these things are pretty rare. So when you do have that point in time, when a customer is actually chosen to interact with you is to maximize it.
And one is, they're obviously like servicing and helping that request, but then having the ability to then go and say, okay We see as well that you haven't adopted this feature, or because you're doing this, you could do X or Y and Israeli trying to increase engagement and just switch the notion of support being a cost driver tool or more of it being a growth driver.
And how can you increase retention, which is a little bit different from this topic. But I like that. There's interesting. You mentioned that too. I think it's definitely an interesting episode for the listeners too. To listen to. Nice. So I see we're running up on time as well. Now I want to save some time for a couple of questions.
I ask every guest, [00:28:00] let's imagine a hypothetical scenario. You arrive at a new company, churn and retention not doing great. This year it comes to you and says, headstart us. Like you really need to turn things around. You've got 90 days to make a dent. What do you do?
Tadas Labudis: [00:28:15] Yeah, I think, I'm going to sound like a repeating record, but I do think you need to find some way to understand what's going on with your customers.
So of course, get the stats on, revenue metrics look at things like ChartMogul or profitable or whatever you have, like where the churn is like, is it out of bounds for a company of that size or that type, and then you need to dig into why people are turning. Whether it's through some interview, someone's done some surveys someone's wrong.
Or, if you just want to reach for some support tickets that maybe mentioned churn as a first point of call any to understand those issues and then recognize, which drivers aren't in your control and which are not, and the things that are not in your control you can't change, but the things that are about the product [00:29:00] feature requests, these are medication can all change.
Yep. Absolutely. I think this is definitely the number one answer that comes back is like understanding your customers going deep into that, because that's really going to inform the strategy and decisions you make. Last question then let's the question that I ask as well, the next is what's one thing that you know today about Chen and retention that you wish you knew when you got started with your career.
I started my career. I didn't even know what turn was. I didn't know what were the percentages of like how to calculate them. So I think maybe it shouldn't be a given that every person in this space understands what it is. I think it's quite specific the SAS and subscription businesses as well.
When I was working mobile we were talking about retention. And there wasn't a point where customers churn. It was just like, did not come back to your product in the last 30 days. And that's like a ghosting kind of effect, but I think maybe there should be more education about what churn is.
And I think podcasts like this really bring it to light and [00:30:00] allow people to understand it in deeper ways. What is good churn, what's bad churn, like how to measure it. What are the pros and cons of those approaches and how to tackle it if it's not where you want it to be? Yeah, I feel like I just didn't know about a concept needed to be sources online.
Now. I feel like I have, there's so
much out there. Very cool, man. Thanks so much for joining today. really appreciate your time. As I mentioned, like they'll obviously be in the show notes is any final thoughts you want to leave the listeners with anything they should be aware of?
Keep up to speed with your work.
Yeah. B do Post some articles on space. So if you're interested in customer feedback analysis, how to understand your customers, what companies do to collaborate better around data. Definitely check out outside blog, outside.com/blog. And also another thing you'd like to recommend is just a book I read actually quite early in my career that I think was transformational.
So probably the shortest book that was the most impactful on me. And it's called the mom test. Bye. I've read it before. The monk test is [00:31:00] essentially a book about what kind of questions you ask and how the questions you ask and have an undesired influence on or could lead your responding to avenues, not giving help in arts for, so this is a book by Rob Fitzpatrick, hold them on test.
I'd definitely recommend to everyone to understand how to do a customer research.
Andrew Michael: [00:31:21] Yeah, absolutely. I think that's also it's the mom test is used in quite a lot of different accelerator programs, as well as the go-to sort of methodology when it comes together in collecting feedback. It's a great recommendation.
We'll add that in the show notes as well for everybody to check out, but again, thank you so much. Wish you best of luck now going forward into 2021.
Tadas Labudis: [00:31:42] Thank you, Andrew was a pleasure being here and thanks so much for your time.
Andrew Michael: [00:31:46] Cheers
Comments
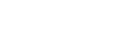
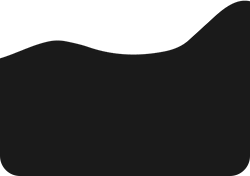
Tadas Labudis
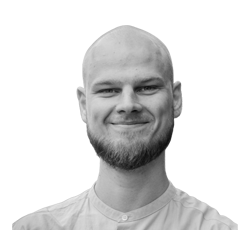
A new episode every week
We’ll send you one episode every Wednesday from a subscription economy pro with insights to help you grow.
About
The show
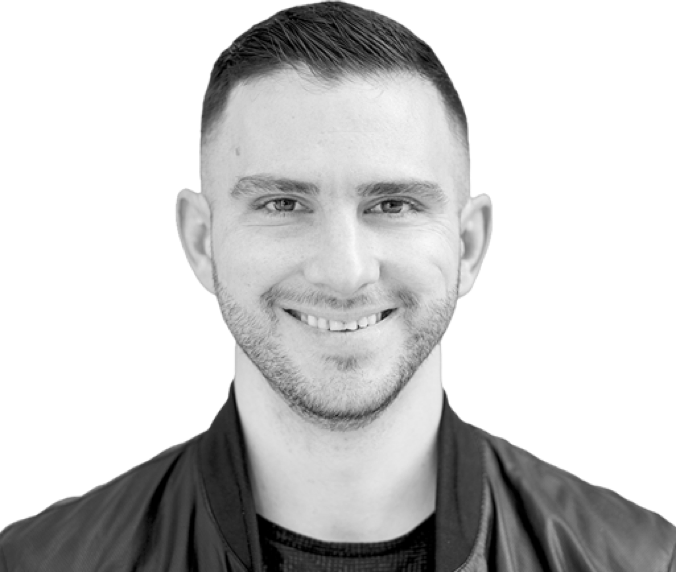
My name is Andrew Michael and I started CHURN.FM, as I was tired of hearing stories about some magical silver bullet that solved churn for company X.
In this podcast, you will hear from founders and subscription economy pros working in product, marketing, customer success, support, and operations roles across different stages of company growth, who are taking a systematic approach to increase retention and engagement within their organizations.